PUBLICATIONS LIST
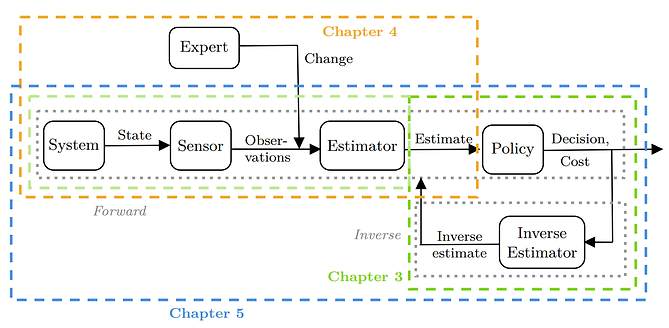
ADVERSARIAL SYSTEMS
We consider sequential stochastic decision problems in which an agent optimizes its local utility by solving a stochastic program and, subsequently, announces its decision to the world. Given its decision, we study the problem of estimating the agent’s private belief (i.e., its posterior distribution over the set of states of nature) based on its private observations. We also consider the counter-adversarial sequential decision-making problem of preventing an adversary from estimating the private belief of the agent.
​
I. Lourenço, R. Mattila, C. R. Rojas, and B. Wahlberg, “Hidden Markov Models: Inverse Filtering, Belief Estimation and Privacy Protection,” Journal of Systems Science and Complexity (JSSC), 2021
​
I. Lourenço, R. Mattila, C. R. Rojas, V. Krishnamurthy, and B. Wahlberg, “How to protect your privacy? A framework for counter-adversarial decision making,” 59th IEEE Conference on Decision and Control (CDC), 2020.
R. Mattila, I. Lourenço, C. R. Rojas, and B. Wahlberg, “What did your adversary believe? Optimal filtering and smoothing in counter-adversarial autonomous systems,” IEEE International Conference on Acoustics, Speech and Signal Processing (ICASSP), 2020.
​
R. Mattila, I. Lourenço, C. R. Rojas, V. Krishnamurthy, and B. Wahlberg, “Estimating private beliefs of Bayesian agents based on observed decisions,” IEEE Control Systems Letters (LCSS), 2019.
​
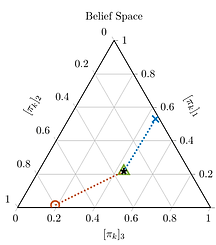
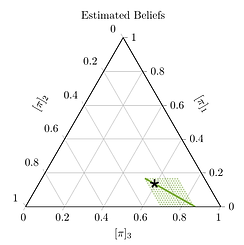
COOPERATIVE SYSTEMS
We consider a cooperative scenario in which an expert agent (teacher) knows a correct, or at least a good, model of the system and aims to assist a learner-agent (student). We propose correctional learning as an approach to the above problem: in order to assist the student, the teacher can intercept the observations collected from the system and modify them to maximize the amount of information the student receives about the system.
R. Winqvist, I. Lourenço, F. Quinzan, C. R. Rojas, and B. Wahlberg, “Optimal transport for correctional learning,” 62nd IEEE Conference in Decision and Control (CDC), 2023
​
I. Lourenço, R. Winqvist, C. R. Rojas, and B. Wahlberg, “A teacher-student framework for online correctional learning,” 61st IEEE Conference in Decision and Control (CDC), 2022
​
I. Lourenço, R. Mattila, C. R. Rojas, and B. Wahlberg, “Cooperative system identification via correctional learning,” 19th IFAC Symposium on System Identification (SYSID), 2021
​


BIOLOGICALLY-INSPIRED SYSTEMS
We consider the problem of replicating a biologically-inspired framework with the aim of obtaining insight about the intrinsic parameters of biological systems. We focus on time perception – how humans and animals perceive the passage of time. We propose a framework that combines internal neuronal mechanisms and external (environmental) stimuli, and implement it in a simulated robot. Next, by analyzing the behavior of agents equipped with the framework, we propose an estimator to infer characteristics of the timing mechanisms intrinsic to the agents.
​
I. Lourenço, R. Mattila, R. Ventura and B. Wahlberg, “A Biologically-Inspired Computational Model of Time Perception,” IEEE Transactions on Cognitive and Developmental Systems (TCDS), 2021.
​
I. Lourenço, R. Ventura, and B. Wahlberg, “Teaching Robots to Perceive Time: A Twofold Learning Approach,” Joint IEEE 10th International Conference on Development and Learning (ICDL), 2020.
​

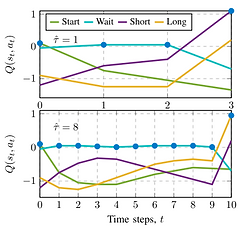
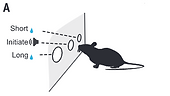
Human-Robot Interaction
Robots have been increasingly better at doing tasks for humans by learning from their feedback, but still often suffer from model misalignment due to missing or incorrectly learned features. When the features the robot needs to learn to perform its task are missing or do not generalize well to new settings, the robot will not be able to learn the task the human wants and, even worse, may learn a completely different and undesired behavior. We propose a framework to separate two sources of misalignment: determining whether
a feature the robot needs is incorrectly learned and does not generalize to new environment setups vs. is entirely missing from the robot’s representation. Once we detect the source of error, we show how the human can initiate the realignment process for the model.
​
I. Lourenço, A. Bobu, C. R. Rojas, and B. Wahlberg, “Diagnosing and augmenting feature representations in correctional inverse reinforcement learning,” 62nd IEEE Conference in Decision and Control (CDC), 2023.
​
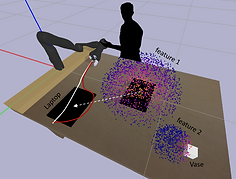
![]() | ![]() | ![]() |
---|
OTHERS
-
Talk on Advanced topics in robotics - Building the future with robots, at University of Rwanda, October 2023
​
-
Talk on Introduction to robotics at Botho University, October 2023
​
-
Poster at WASP@ISAB - WASP International Scientific Advisory Board, August 2023
​
-
Talk at OsloMet about Neuroscience for AI and robotics, June 2023
​
-
Talk at Thai embassy event about Digitalization, May 2023
​
-
Learning from interactions. Poster at WASP Winter Conference, January 2023
​
-
Learning from interactions. Poster at WASP study trip to the UK, October 2022
​
-
Model Misalignment in Human-Robot Interaction. Poster at ERNSI, September 2022
​
-
Talk at Iliad group, Stanford University, July 2022
​
-
Inês Lourenço and Rebecka Winqvist, A teacher-student framework for online correctional learning. Poster at the 2022 WASP Winter Conference, January, online.
​​
-
Inês Lourenço, Robert Mattila, Cristian R. Rojas and Bo Wahlberg, Correctional learning for cooperative system identification. Poster at the 2021 Workshop of the European Research Network on System Identification (ERNSI), online.
​​​
-
Inês Lourenço and Bo Wahlberg, Learning dynamical systems. Poster at the 2019 WASP Winter Conference, January, Gothenburg, Sweden.
​
-
Robert Mattila, Inês Lourenço, Cristian R. Rojas, Vikram Krishnamurthy and Bo Wahlberg, Estimating Private Beliefs Based on Observed Decisions. Poster at the 2019 Workshop of the European Research Network on System Identification (ERNSI), September, Maastricht, the Netherlands.​
​​
-
Talk at the 2018 Workshop of the European Research Network on System Identification (ERNSI), September, Cambrige, UK.
Teaching and Supervision
-
Teaching assistant for the Reinforcement Learning course EL2805, 2022
-
Teaching assistant for the Automatic Control course EL1000, 2019, 2020, 2021
-
Master thesis supervisor, in collaboration with CLIMEON, Spring, 2023​​
-
Lab responsible, 2021-2022
-
Bachelor thesis supervisor, Spring, 2020
-
Bachelor thesis supervisor, Spring, 2019